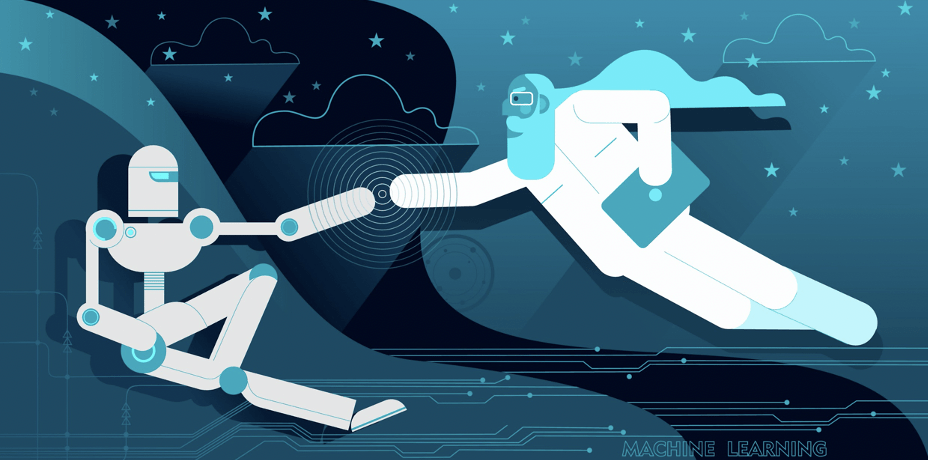
An Introduction to Ethics in AI
Background of Artificial Intelligence
Artificial Intelligence (AI) has been a hot topic in the twenty-first century. It’s become so prevalent that there’s a need for over a million AI engineers worldwide, YouTube created a nine-video series on AI, and Elon Musk started a company called Neuralink in response to his concerns around AI. AI has almost doubled in interest over the past five years according to Google Trends, but has been around since the 1950’s — Norbert Wiener theorized that all intelligent behavior was the result of feedback mechanisms and this very idea influenced much of the early development of AI. However, for reasons which I’ll explain below, the emergence of AI and its potential is relatively new.
The truth is that artificial intelligence and the ideas that surround it are fairly misunderstood, and I believe that the lack of understanding around AI is a factor in why it’s such a controversial topic. In fact, controversies are thought to be a result of a lack of confidence on the part of the disputants. For example, in regard to controversies over climate change, it’s been proposed that some people are opposed to the scientific consensus because they don’t have enough information about the topic.
Also, it’s worth noting that by no means am I an expert either. However, by writing this, I hope to achieve a few goals:
1. I want to personally develop a better understanding of AI by researching and writing this essay.
2. I want to provide a resource that can help make the general population more informed about artificial intelligence and its potential implications.
3. Most importantly, I hope that this essay generates open discussions about AI so that we can collectively critically think about how AI will shape humanity’s future.
What is Artificial Intelligence?
According to Techopedia, “Artificial Intelligence (AI) is a branch of computer science that aims to imbue software with the ability to analyze its environment using either predetermined rules and search algorithms, or pattern recognizing machine learning models, and then make decisions based on those analyses.”
Textbooks define this field as the study of intelligent agents, defined as any device that perceives its environment and takes action to maximize its chance of successfully achieving its goals.
Why is Artificial Intelligence so popular now?
So if the idea of AI has been around for several decades, why is it only now that it’s gaining so much traction? A few reasons:
Quantity of Data: Perhaps the main reason why AI is becoming so popular is due to the sheer amount of data that’s been accumulated since the tech bubble. Typically, in order for AI applications, like neural networks [2], to perform well, they need to have a considerable amount of data. In 1995, less than 10% of the world population used the Internet. Now, internet users make up 58% of the global population and generate over 2.5 quintillion [3] bytes of data a day. With data scarcity no longer being an issue, it has opened the potential of developing actual AI applications.
Computing Power: The second biggest reason why AI has gained traction recently is due to improvements in computer power. Mainly, the increase in the power of graphical processing units (GPUs) has allowed organizations to run massive neural network applications at speeds that were not possible to hit a decade ago.
Other reasons include the availability of inexpensive cloud storage and the developments in software for deep learning and its open-source availability.
The Fears and Concerns Surrounding Artificial Intelligence
Because AI is such a new topic, it has not only spurred a lot of controversies, but also speculation, skepticism, and concerns.
This leads us to the most popular hypothesis, technology singularity, which is the idea that there could be a point in time at which technological growth becomes uncontrollable and irreversible, resulting in unforeseeable changes to human civilization. The singularity is expected to occur after the time when technological creations exceed the computing power of human brains. Ray Kurzweil, an inventor and futurist, predicts that based on Moore’s Law and the general trend of exponential growth, that the singularity will come before the mid-21′ st century. Moore’s Law states that we can expect the speed and capability of our computers to increase every couple of years and we will pay less for them.
More specific and more relevant than the idea of technological singularity is a theory called Intelligence Explosion, an upgradable intelligent agent that will eventually enter a runaway reaction of self-improvement cycles, resulting in a powerful superintelligence that qualitatively surpasses all human intelligence. Superintelligence is a hypothetical agent that possesses intelligence far surpassing that of the brightest and most gifted human minds. This is predicated on the idea of artificial general intelligence (AGI), which is the hypothetical intelligence of a machine that has the capacity to understand or learn any intellectual task that a human being can.
What might be more realistic and perhaps more of an immediate issue is the bias in AI systems. Bias is the prejudice in favor of or against one thing, person, or group compared with another, usually in a way considered to be unfair. Referring back to the definition of artificial intelligence, it aims to imbue software with the ability to analyze its environment using either predetermined rules and search algorithms or pattern recognizing machine learning models. These rules, algorithms, and models may have underlying biases that stem from the data in which it was created or the engineers that developed the system.
This is dangerous because it can result in privacy violations, discrimination, and serious social consequences. For example, Amazon’s machine learning algorithm for recruiting was found to be biased against women. The reason for that was because the algorithm was based on the number of resumes submitted over the past ten years, and since most of the applicants were men, it was trained to favor men over women.
In addition to bias in AI are a number of other potential risks, including privacy invasion and malicious intent. Privacy invasion occurs when personal information is being collected that we have not given consent to. The availability of too much personal information can pose social risks. Malicious intent refers to creating AI systems to do harm to society. An example of this is creating military weapons that use AI to detect a person of a specific race, ethnicity, etc…
Combining Ethics and Artificial Intelligence
As a response to the risks of AI, many organizations have launched various initiatives to establish ethical principles for the adoption of socially beneficial AI. Luciano Floridi, a Professor of Philosophy and Ethics of Information at the University of Oxford, analyzed several of the highest-profile sets of ethical principles for AI and developed an overarching framework of five core principles for ethical AI, four of which are commonly used in bioethics, the study of the ethical issues emerging from advances in biology and medicine.
The Five Principles of Ethical AI are as follows:
1. Beneficence
Beneficence refers to promoting well-being, having a positive benefit on humanity, and sustaining the planet. The Montreal Declaration for Responsible AI said in regards to beneficence that “the development of AI should ultimately promote the well-being of all sentient creatures.”
2. Non-maleficence
Non-maleficence means to do no harm or inflict the least possible harm to reach an outcome. Do not mistake this for beneficence — “doing good” and “doing no harm” are two different things. Of particular concern is the prevention of infringements on personal privacy.
3. Autonomy
By implementing AI systems, we as humans willingly give up some of our decision-making power. However, the principle of autonomy means to strike a balance between the decision-making power we retain for ourselves and that which we delegate to artificial agents. The Montreal Declaration stated that “the development of AI should promote the autonomy of all human beings.”
4. Justice
Justice means to preserve solidarity and avoid unfairness — it is the solution to bias in AI. The Montreal Declaration specifically defines this principle as so: “the development of AI should promote justice and seek to eliminate all types of discrimination.”
5. Explicability
Lastly is explicability, which refers to the need to understand and hold to account the decision-making processes of AI. We need to promote transparency and interpretability when it comes to AI and hold people accountable for their actions.
If the framework above provides a coherent and sufficiently comprehensive overview of the central ethical principles for AI (Floridi et al., 2018), then it can serve as the architecture within which laws, rules, technical standards, and best practices are developed for specific sectors, industries, and jurisdictions.
Where do we go from here?
The truth is that I don’t really know.
But what I know for sure is that this needs to be an ongoing discussion. We need to bring this to people’s attention so that they’re at least aware of the 5 principles, especially those that work with machine learning models and AI systems.
How can we eliminate all harmful biases in AI? What is considered to be of beneficence? What are the boundaries of what’s good and bad? How can we hold people accountable for their actions? Are these principles enforceable? If not, what is? If so, how can we enforce these?
Thanks for Reading!
Here at Datatron, we offer a platform to govern and manage all of your Machine Learning, Artificial Intelligence, and Data Science Models in Production. Additionally, we help you automate, optimize, and accelerate your ML models to ensure they are running smoothly and efficiently in production — To learn more about our services be sure to Book a Demo.