AI Governance: Artificial Intelligence Model Governance
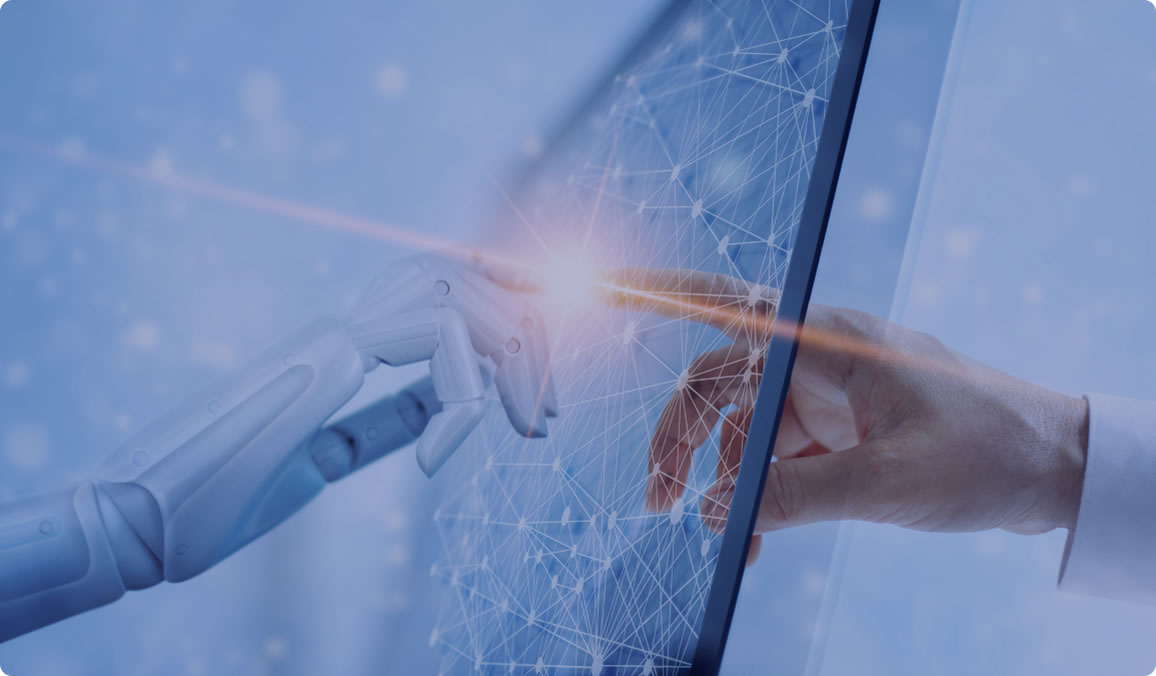
What is AI governance? At the federal level (e.g., United States government), Artificial intelligence governance is the idea of having a framework in place to ensure machine learning technologies are researched and developed with the goal of making AI system adoption fair for the people. While we’ll be focusing on the corporate implementation of AI Governance in this article, it is essential to understand AI governance from a regulatory perspective, as laws at the governmental level influence and shape corporate AI governance protocols.
AI governance deals with issues such as the right to be informed and the violations that may occur when AI technology is misused. The need for AI governance is a direct result of the rise of artificial intelligence use across all industries. The healthcare, banking, transportation, business, and public safety sectors already rely heavily on artificial intelligence.
The primary focus areas of AI governance are how it relates to justice, data quality, and autonomy. Navigating these areas requires a close look at which sectors are appropriate and inappropriate for artificial intelligence and what legal structures should be involved. AI governance addresses the control and access to personal data and the role morals and ethics play when using artificial intelligence.
Ultimately, AI governance determines how much of our daily lives can be shaped and influenced by AI algorithms and who is in control of monitoring it.
In 2016, the Obama Administration announced the White House Future of Artificial Intelligence Initiative. It was a series of public engagement activities, policy analysis, and expert convenings led by the Office of Science and Technology Policy to examine the potential impact of artificial intelligence.
The next five years or so represent a vital juncture in technical and policy advancement concerning the future of AI governance. The decisions that government and the technical community make will steer the development and deployment of machine intelligence and have a distinct impact on how AI technology is created.
In this article, we’re going to focus on AI governance from the corporate perspective and see where we are today with the latest AI governance frameworks.
Table of Contents
Why Do We Need AI Governance?
Who is Responsible for Ensuring AI is Used Ethically?
What are the 4 Key Principles of Responsible AI?
How Should AI Governance be Measured?
What are the Different Levels of AI Governance?
Why You Should Care About AI Model Governance?
What To Look For In An AI Governance Solution?
Model Governance Checklist
Your AI Program Deserves Liberation.
Datatron is the Answer.
Why Do We Need AI Governance?
To fully understand why we need AI governance, you must understand the AI lifecycle. The AI lifecycle includes roles performed by people with different specialized skills that, when combined, produce an AI service.
Each role contributes uniquely, using different tools. From origination to deployment, generally, there will be four different roles involved.
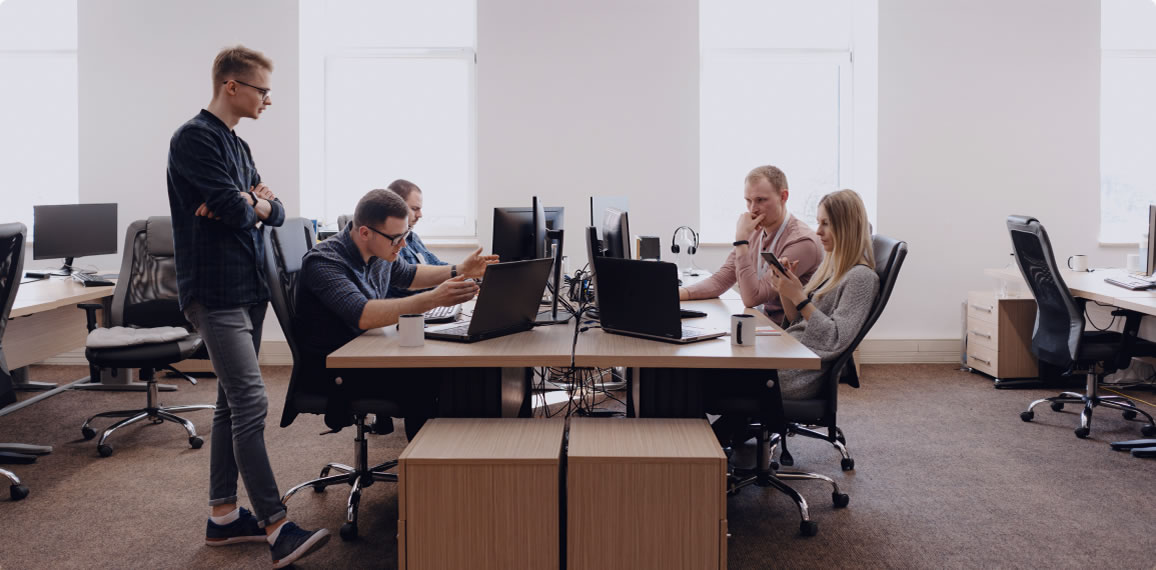
Business Owner
The process starts with a business owner who defines a business goal and requirements to meet the goal. Their request will include the purpose of the AI model or service, how to measure its success, and other constraints such as bias thresholds, appropriate datasets, and levels of explainability and robustness required.
The Data Scientist
Working closely with data engineers, the data scientist takes the business owner’s requirements and uses data to train AI models to meet the requirements. The data scientist, and expert in computer science, will construct a model using a machine learning process. The process includes selecting and transforming the dataset, discovering the best machine learning algorithm, tuning the algorithm parameters, etc. The data scientist’s goal is to produce a model that best satisfies the business owner’s requirements.
Model Validator
The model validator is an independent third party. This role falls within the scope of model risk management and is similar to a testing role in traditional software development. A person or company in this role will apply a different dataset to the model and independently measure metrics defined by the business owner. If the validator approves the model, it can be deployed
AI Operations Engineer
The artificial intelligence operation engineer is responsible for deploying and monitoring the model in production to ensure it operates as designed. This may include monitoring the performance metrics as defined by the owner. If some metrics are not meeting expectations, the operations engineer is responsible for informing the appropriate roles.
With so many roles involved in the AI lifecycle, we need AI governance to protect the companies using AI solutions in emerging technologies and to protect the consumers using AI technologies across the entire global community.
Who is Responsible for Ensuring AI is Used Ethically?
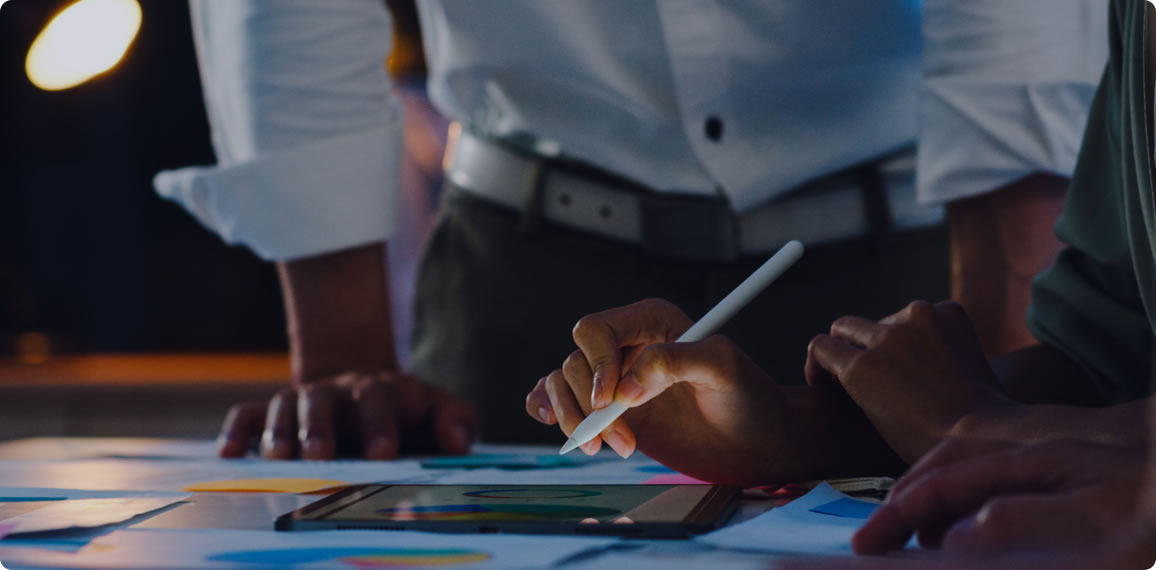
With the number of roles involved in the AI lifecycle, a question arises: who should be responsible for AI governance?
First, CEOs and senior leadership in corporate institutions are the people ultimately responsible for ethical AI governance. Second in line in terms of responsibility comes the board of an organization who is responsible for audits.
The general counsel should have the responsibility for legal and risk aspects. The CFO should be aware of the cost and financial risk elements. The chief data officer (CDO) should take responsibility for maintaining and coordinating an ongoing evolution of the organization’s AI governance.
With data critical to all business functions, customer engagement, products, and supply chains, every leader needs to be knowledgeable about artificial intelligence governance. Without clear responsibilities, no one is accountable.