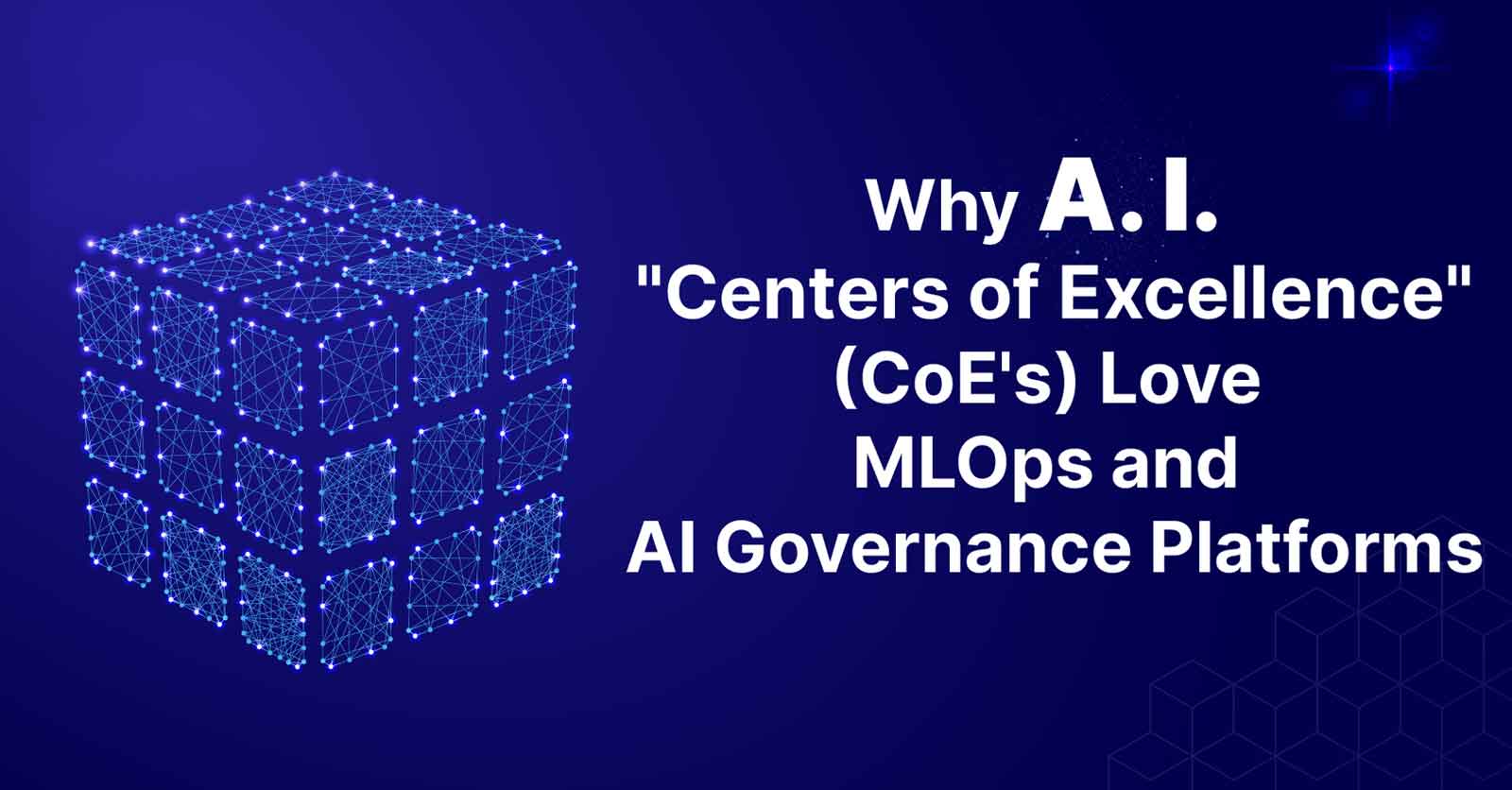
Why AI “Centers of Excellence” (CoE’s) Love MLOps and AI Governance Platforms
Artificial Intelligence is commonly accepted as the fifth pillar of Digital Transformation. While AI is in its nascent stages, larger enterprises have already begun to see the promise of AI and nucleated data science teams to experiment and map initiatives to business objectives. Even “mature” AI programs are circling back to correct foundational issues. With early success comes growth and growing pains, and CoE’s are coming to the rescue leveraging MLOps and AI Governance platforms which remedy accumulated pain points from in-house built solutions, which is where most AI programs start. Here’s what CoE’s appreciate about MLOps and AI Governance platforms, pain points they solve, and what to look for when selecting a an AI platform.
-
A Scalable Standard
As AI first makes an appearance in the enterprise and shows promise, word spreads fast, and different business units (BUs) begin to build and grow their own AI/ML practice. Teams share learnings but employ tools and teams that are redundant. Growing pains include lots of manual work to get even a single model into production and deliver value to the business. Then sprinkle in human attrition as your most valuable data science resources, your data scientists, jump ship to competitors or FAANGs, taking with them the institutional knowledge, and worse, the know-how of solutions that were home-built. AI executives are then forced to start over, thus slowing the ROI of AI. Implementing a standard platform ensures that expertise, performance, and value remain when employee turnover is a constant.
-
Enterprise-wide
While sibling teams, business units (BU), and lines of business (LOB) each build their own AI program to suit their needs, redundancy proliferates and it usually takes an overarching organization within the organization to streamline AI. Enter the “Center of Excellence,” a wellspring of wisdom, collaboration, and subject matter expertise. While no one likes being forced to color between the lines when previously allowed to run free-range, eventually, all stakeholders understand and appreciate at least some of the benefits of a central governing body to support the proliferation and success of AI in the enterprise. An enterprise-wide platform with de-coupled MLOps and Governance features allows varying business entities to adopt the platform at a pace and implementation that suits their particular needs. Think you have MLOps solved? Great, just use the Governance features (then see #4 below for a dose of harsh reality). Still early in your group’s AI lifecycle? Just use the MLOps features to accelerate the heavy lifting of model deployment. Just like you let your data scientists use the best tools for building the best models, allow each group to self-select their AI maturation workflow with a flexible AI platform.
-
Manpower Multiplier
Now that a standard has been defined by the enterprise, processes and teams are put in place to operationalize ML models and accelerate model deployment velocity. While many organizations take three, six, or even twelve months to put a single model into production, with a robust AI platform even small teams can achieve a 10x in AI performance or model deployment velocity like Domino’s did. Additionally, eliminating redundant headcount and fostering deep specialization so that data scientists are no longer required to moonlight as ML Engineers to get their models into production, which takes time away from what they should be doing, which is building new models to generate business value (e.g. profits, time savings, efficiencies). Any AI/ML platform worth a dime will allow a single ML Engineer to deploy, manage, and monitor at scale, supporting multiple BUs and hundreds to thousands of models without breaking a sweat.
-
Deploy Faster
Ask any AI group and they’ll tell you that they have MLOps (Machine Learning Operations) figured out. However, either time or asking the right questions surfaces serious flaws in their “homegrown” MLOps solution. It could be built upon open source tools or libraries that don’t have expedient support options or take an inordinate amount of time and effort to update and maintain. No one likes their baby called ugly, and eventually they realize the “kludge” they’ve built has reached its limit. Perhaps it was the growing time requirement or institutional knowledge walking out the door and constant starting over that caused this self-reflection from leadership. Or, perhaps their internal customer, the data scientists have had enough excuses from DevOps/IT on why their models are not successfully running in production. Regardless, whether self-driven or mandated by a higher-up (more likely), it shows maturity to drive the uncomfortable change necessary to support AI growth in the enterprise – sometimes a step back is required to take two steps forward. A nimble MLOps platform can get a model in production in days, not months, and automates a lot of the manual, repetitive work, that was once spread across BUs, and is now centrally facilitated. What was once a practice in DIY repetition handcuffed by human-power, is now streamlined, repeatable, and scaleable.
-
Responsible, Explainable, and Reliable AI – Governance
Mature AI programs that are delivering value across the enterprise are soon, if not already, encountered by “Risk & Compliance” teams requesting audit reports. AI practitioners at every level and stage must now be seasoned in the “Three Lines of Defense in AI” to fully understand what their models are doing in production for the safety of the consumer, business, and future of AI. In layperson’s terms “Explainable AI” is the concept that you undeniably know what your models are doing and fully understand how they are performing. A proper AI governance platform will include features like a model catalog with version registry and user access controls, all necessary to document what changes were made to a model, who made those changes, and when. Next, it should monitor model features for bias, drift, performance anomalies, and ideally, business KPIs. If a model goes out of predefined “control” thresholds, then the proper team members will be notified. Most importantly, the platform should provide reporting that helps data scientists discover the root cause of the issue or at least provide directional cues so that they can remedy the issue. Mature AI platforms will support each of the three “Lines of Defense” and deliver audit reports that satisfy Risk & Compliance requirements.
As an Artificial Intelligence “Center of Excellence” and “Digital Transformation” executive, you know the challenges at the human, group, and organizational level of supporting AI. While the greatest challenge may be educating very, very, VERY smart stakeholders that there is a better way forward that involves change, and that no solution will 100% solve every stakeholder’s most pressing needs, the AI platform you select should address the aforementioned pain points in the least. In doing so, you guarantee that the most banal functions are removed as barriers, human talents and model value are multiplied, while institutional knowledge is retained and built upon resulting in more ROI from AI.
The Datatron is an AI/ML platform with discrete MLOps and AI governance solutions that help companies generate more ROI from their program faster, while ensuring they are doing so responsibly. Available for cloud or on-premise, Datatron’s model catalog, bias, drift monitoring, and MLOps features empower organizations to graduate more models to production, quicker, and see an ROI on their ML program up to 80% faster than open source and homegrown solutions. The AI governance features, including the “Health” dashboard, monitoring, alerts, and reporting provide “explainability” and support risk and compliance requirements. Leading brands including Domino’s Pizza and Comcast rely on the Datatron to operationalize and govern AI models at scale. Founded in 2016, Datatron is a privately held, venture-backed company headquartered in San Francisco, Calif. Learn more and request a Demo at https://www.datatron.com/request-a-demo/