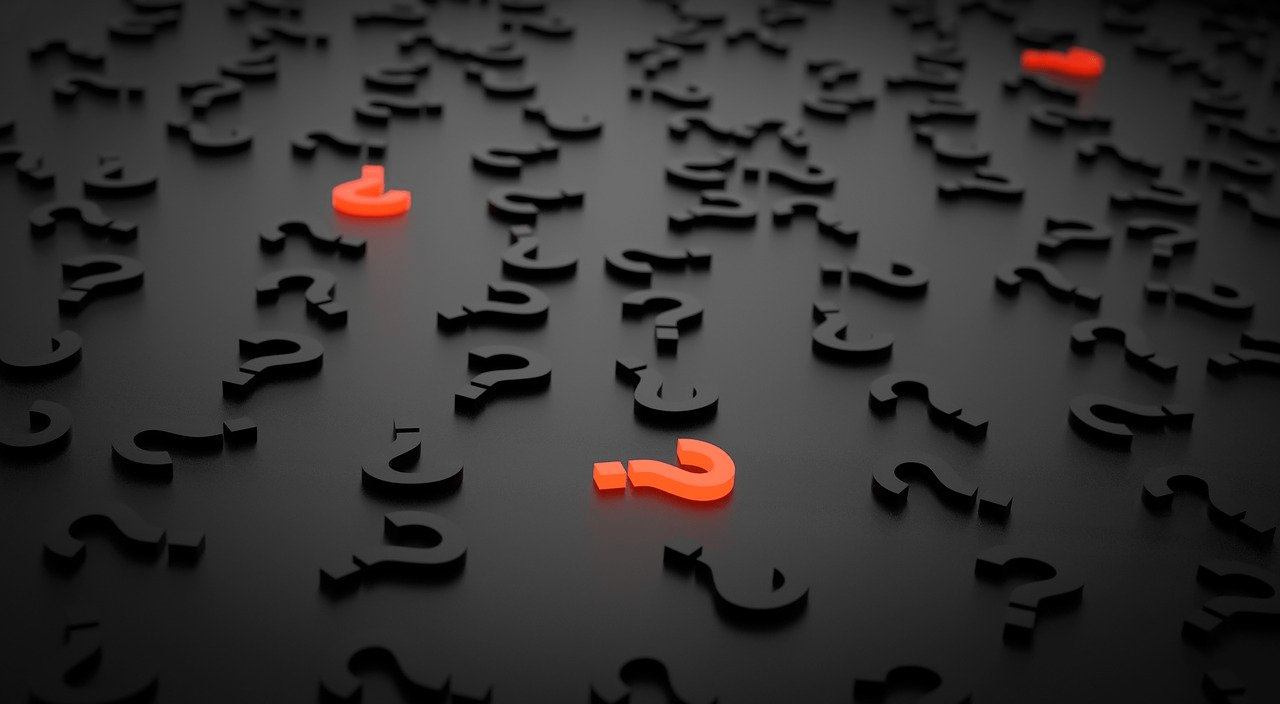
What is Statistical Bias and Why is it so Important in Data Science?
Image by Arek Socha from Pixabay
Introduction
Imagine this.
You’re running for president and you want to be the voice of the majority.
So you head to an environmentalist movement and ask five people what they think about the meat industry and all five of them unanimously say that meat production should be banned. Immediately, you’re convinced that everyone wants to ban meat production to save Earth.
You make this your headline for your campaign and preach it day and night thinking that this is the secret to winning your campaign.
4 months later, you end up with less than 1% of the votes.
Your idiocracy could have easily been avoided should you have known about bias.
Bias is important, not just in statistics and machine learning, but in other areas like philosophy, psychology, and business too.
Generally, bias is defined as “prejudice in favor of or against one thing, person, or group compared with another, usually in a way considered to be unfair.”
Bias is bad. We want to minimize as much bias as we can.
What is statistical bias?
For this article, we’re going to focus on statistical bias. Statistical bias is essentially when a model or statistic is unrepresentative of the population, and there are several sources of bias that cause this.
Types of statistical bias
The most common sources of bias include:
- Selection bias
- Survivorship bias
- Omitted variable bias
- Recall bias
- Observer bias
- Funding bias
Selection bias
Selection bias is the phenomenon of selecting individuals, groups, or data for analysis in such a way that proper randomization is not achieved, ultimately resulting in a sample that is not representative of the population. [1]
Within selection bias, there are several types of selection bias:
- Sampling bias: refers to a biased sample caused by non-random sampling.
To give an example, imagine that there are 10 people in a room and you ask if they prefer grapes or bananas. If you only surveyed the three females and concluded that the majority of people like grapes, you’d have demonstrated sampling bias.
- Time interval bias: bias caused by intentionally specifying a certain range of time to support the desired conclusion. For example, concluding the average number of tweets per hours from a sample taken from peak hours (9–12AM) is an example of time interval bias.
Taken from Buffer.com, referenced below
- Susceptibility bias: includes clinical susceptibility bias, protopathic bias, and indication bias, which all relate to the idea of potentially mixing up cause and effect and correlation.
- Confirmation bias: the tendency to favour information that confirms one’s beliefs.
Taken from John Cook, referenced below
Survivorship bias
The phenomenon where only those that ‘survived’ a long process are included or excluded in an analysis, thus creating a biased sample.
A great example provided by Sreenivasan Chandrasekar is the following:
“We enroll for gym membership and attend for a few days. We see the same faces of many people who are fit, motivated and exercising everyday whenever we go to gym. After a few days we become depressed why we aren’t able to stick to our schedule and motivation more than a week when most of the people who we saw at gym could. What we didn’t see was that many of the people who had enrolled for gym membership had also stopped turning up for gym just after a week and we didn’t see them.”
Omitted variable bias
This is bias that stems from the absence of relevant variables in a model. In machine learning, removing relevant and/or too many variables results in an underfit model.
An example of this is purchasing a car based on the brand and the car model, but not the mileage. Imagine a 2020 Porsche 911 turbo for $10,000 — sounds like a steal until you find out that there’s 400,000 miles on it.
Recall bias
Recall bias is a type of information bias where participants do not ‘recall’ previous events, memories, or details.
This is also related to recency bias, where we tend to remember things better that have happened more recently.
Observer bias
This is the bias that stems from the subjective viewpoint of observers and how they assess subjective criteria or record subjective information.
Funding bias
Also known as sponsorship bias, it is the tendency to skew a study or the results of a study to support a financial sponsor.
For more articles like this one, check out https://datatron.com/blog
Here at Datatron, we offer a platform to govern and manage all of your Machine Learning, Artificial Intelligence, and Data Science Models in Production. Additionally, we help you automate, optimize, and accelerate your ML models to ensure they are running smoothly and efficiently in production — To learn more about our services be sure to Book a Demo.