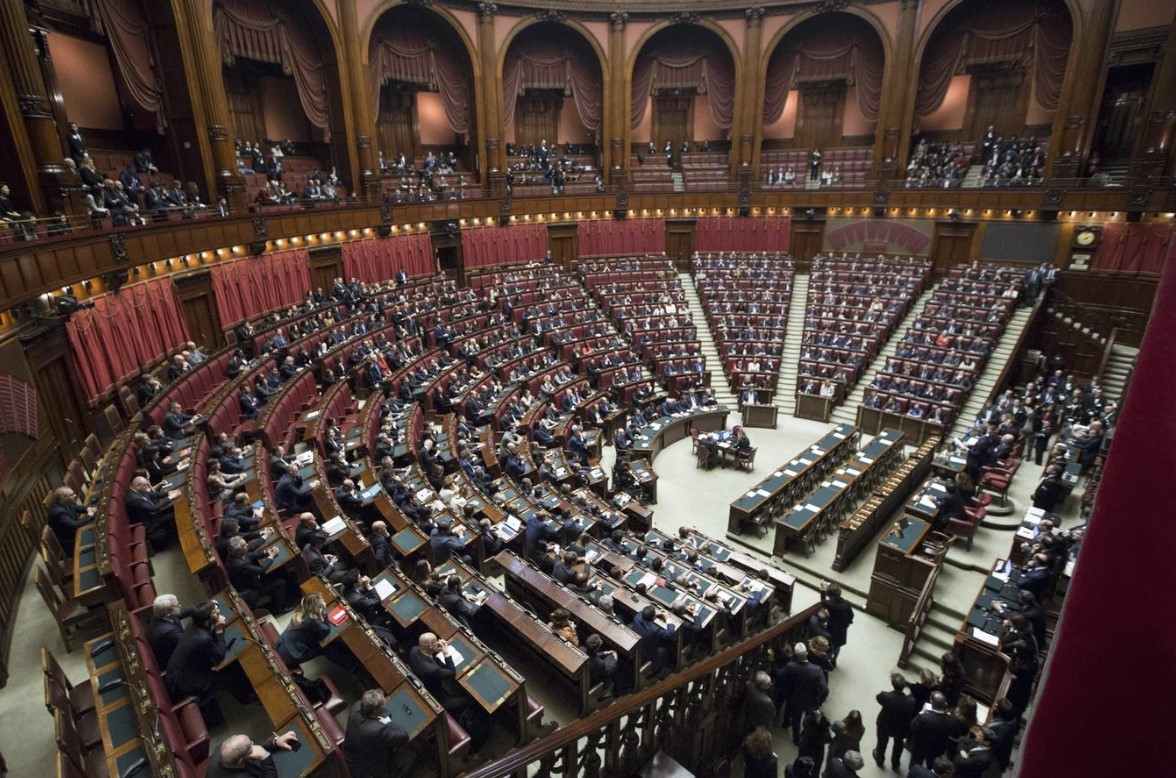
Model Governance Applied To Finance
Machine learning is a subfield of the broader Artificial Intelligence field. It explores algorithms that provide systems with the ability to learn and improve automatically from experience without having to be explicitly programmed. The majority of modern applied Artificial Intelligence relies heavily on some sort of Machine Learning algorithm being used. Consequently, the terms Machine Learning (ML) and Artificial Intelligence (AI) have been used interchangeably in business, hence we would also use them interchangeably in this post.
It is no secret that the adoption of Artificial Intelligence in production is increasing rapidly across multiple industries with the early adopters being recorded within financial industries. As Artificial intelligence matures simultaneously with the application of technology in business, we can expect to reach solutions to problems that were in the past unaddressable since they were beyond human capabilities, as well as those of a computer – for example, it would be extremely difficult to write set of heuristics that track the market flows, and/or deny people credit as these feats are constantly changing. Also, we can expect to experience advancements in efficiency because of the innate automatic learning and improvement that AI unlocks.
However, alongside the impeccable capabilities of AI comes the increased impact of AI-specific risk. There are a number of specific risks presented by AI which must be managed since Artificial Intelligence introduces a new dimension for model risk management, though it’s important to state that good model governance concepts are industry agnostic and the best practices can be applied to a wide spectrum of other domains.
Image by @marcooriolesi on Unsplash
How AI is Impacting Financial Services
Financial Services is one of the areas in industry where Artificial Intelligence is prevalent beyond the proof-of-concept (POC) or exploration stages of development. Considering AI technology often tends to build on traditional statistical modeling techniques – such as Linear Regression models, Autoregression models, and Moving Average models – and generally is more much more powerful than its close relative, they are often used in finance to complement the traditional methods although occasionally they may be an outright substitution for them.
Additionally, the great power of AI has also unlocked much more potential to bring added value in new areas which the conventional models were insufficient. To name of a few:
- Voice Assistance
- Brand Sentiment Analysis
- Credit Scoring
- Document Search
However, to crack down on the risk brought about from the wide use of and potential impact of model-based decision making, the sector has kept in check with extremely strict and comprehensive regulatory scrutiny regarding Model Risk Management (MRM). This in-turn has resulted in the industry as a whole gaining bags of experience in MRM, hence developing a regulator-driven MRM framework better known as SR 11-7 in the US.
Before the introduction of SR 11-7, most banks would conduct their own form of validation. SR 11-7 generalized the expectations of banks using model-based decision making and introduced the idea of model risk as a risk that ought to be managed like risk would be. Consequently, it is quite commonplace for large banks to have designed and implemented MRM frameworks that are consistent with the regulations since many other regulators began to issue guidance with a consistent approach to MRM.
The Elements of MRM Frameworks
As a result of the introduction of generalized regulation, MRM has developed into a very mature discipline that must be followed by players within the financial sector. Though frameworks may vary between banks, there are some key elements that tend to remain consistent across the board such as the following:
Developer Testing and Detailed Documentation – It is the responsibility of the model developer to provide clear and concise reasoning for the methods they’ve decided to proceed with, as well as evidence that the model has been tested before it is deployed to a live environment. Encompassing this responsibility includes well-written model documentation that details technical information and the key assumptions that the model makes.
Review and Validation – There should also be an independent party that is capable and thereby responsible for reviewing and validating all components of the model.
The two validation stages presented above may be thought of as the 1st and 2nd lines of validation; The key behind these teams is to focus on how conceptually sound the model behaves. This includes explaining model assumptions and model limitations, considering the possible benefits and disadvantages of different ways of approaching the solution and conducting thorough tests of the model’s behavior (i.e. stress testing, limiting cases, etc.)
Periodic Reviews – Once the model is in production, periodic reviews and continuous model performance monitoring is imperative to ensure the model is continuing to perform intended, and that the most recent validation is still a reliable indicator.
Model Inventory – The most important information regarding each model must be kept in a database that can be accessed via an inventory tool. The central principle of any functioning MRM framework would consist of an up-to-date inventory tool that will process the model approval workflow then aggregate the model information into model risk reports.
Model Risk Reports – This involves having an effective model committee where similar models are grouped and approved, and model concerns may be discussed in a formal manner with stakeholders. Additionally, this serves as a great channel to continue to report model risk metrics to senior officers (Note: It is imperative that senior officers within an organization are aware of the main risk a model poses.)
Following the 2 validation stages mentioned above comes the 3rd line of validation which is an internal audit. The role of this audit is to ensure that MRM is being approached consistently and effectively across the entire firm.
These elements act jointly to ensure models are used properly with a good intuition surrounding the limitations of the model, minimize the number of modeling errors, and set a high standard for modeling across the firm.
Here at Datatron, we offer a platform to govern and manage all of your Machine Learning, Artificial Intelligence, and Data Science Models in Production. Additionally, we help you automate, optimize, and accelerate your ML models to ensure they are running smoothly and efficiently in production — To learn more about our services be sure to Book a Demo.
Thank you for reading! This blog post was written by Kurtis Pykes. Connect with me to learn to read more Data Science and Artificial Intelligence related articles.