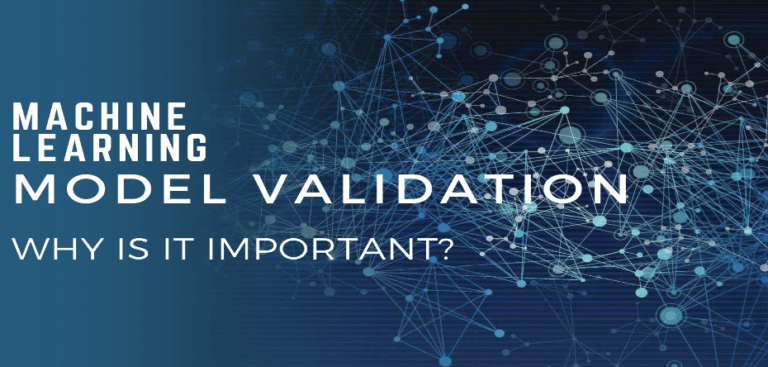
What is Model Validation and Why is it Important?
We all have pursued enough articles about Machine Learning, and the first notion we often come up with is ‘Machine Learning is about making predictions.’
Yes, it is somewhat convincing, but these predictions come up after assorted processes like Data Preparation, Choosing a Model, Training the Model, Parameter Tuning, Model Validation, etc. So, only after carrying out the aforementioned operations, a Machine Learning Model (Regression or Classification) is efficient to make predictions.
Let’s have a look below to have a better understanding.
What is Model Validation?
So, as the name suggests ‘Model Validation’, we can perceive that the model is seeking some validation, but what’s that validation all about? Let’s try to answer it.
Model validation is the process that is carried out after Model Training where the trained model is evaluated with a testing data set. The testing data may or may not be a chunk of the same data set from which the training set is procured.
To know things better, we can note that the two types of Model Validation techniques are namely,
- In-sample validation – testing data from the same dataset that is used to build the model.
- Out-of-sample validation – testing data from a new dataset that isn’t used to build the model
Conclusion alert! Model validation refers to the process of confirming that the model achieves its intended purpose i.e., how effective our model is.
But how is it achieved? Take a look below.
The ultimate goal for any machine learning model is to learn from examples in such a manner that the model is capable of generalizing the learning to new instances which it has not yet seen. So, when we approach a problem with a dataset in hand, it is very important that we find the right machine learning algorithm to create our model. Every model has its own strengths and weaknesses. For instance, some algorithms have a higher tolerance for small datasets, while others may be good with large amounts of data. For this reason, two different models using similar data can predict different results with different degrees of accuracy and hence model validation is required.
Following is the chronology for Model Validation-
-Choose a machine learning algorithm.
-Choose hyperparameters for the model.
-Fit the model to the training data.
-Use the model to predict labels for new data.
Note- In machine learning, we use the term parameters to refer to something that can be learned by the algorithm during training and hyperparameters to refer to something that is passed to the algorithm.
Then the accuracy score for the model is calculated and if in any case, this accuracy score is low, we change the value of the hyperparameters used in the model, and retest it until we get a decent accuracy score.
There are various ways of validating a model among which the two most famous methods are Cross Validation and Bootstrapping but there is no single validation method that works in all scenarios. Therefore, it is important to understand the type of data we are working with.
Although you can read more compositions to learn these techniques better.
Importance of Model Validation
Now after having a glimpse of Model Validation, we all can imagine how important a component it is of the entire Model development process. Validating the machine learning model outputs are important to ensure its accuracy. When a machine learning model is trained, a huge amount of training data is used and the main aim of checking the model validation provides an opportunity for machine learning engineers to improve the data quality and quantity. As it happens, without checking and validating the model it is not right to rely on its prediction. And in sensitive areas like healthcare and self-driven vehicles, any kind of mistake in object detection can lead to major fatalities due to wrong decisions taken by the machine in real-life predictions. And validating the ML model at the training and development stage helps to make the model make the right predictions. Some added advantages of Model Validation are as follows.
- Scalability and flexibility
- Reduce the costs.
- Enhance the model quality.
- Discovering more errors
- Prevents the model from overfitting and underfitting.
It is extremely important that data scientists validate machine learning models that are under training for accuracy and stability as it needs to be ensured that the model picks up on most of the trends and patterns in the data without incurring too much noise.
Now we are clear with the fact that building the machine learning model is not just enough to rely on its predictions, we need to check the accuracy and validate the same to ensure the precision of results given by the model and make it usable in real-life applications.
We, at Datatron, provide an enterprise-grade platform that helps you to supervise your Machine Learning models for high precision deployment to meet the regulatory requirements and effective management of the entire production machine learning life cycle.
Follow us on Twitter and LinkedIn.
Thanks for reading!