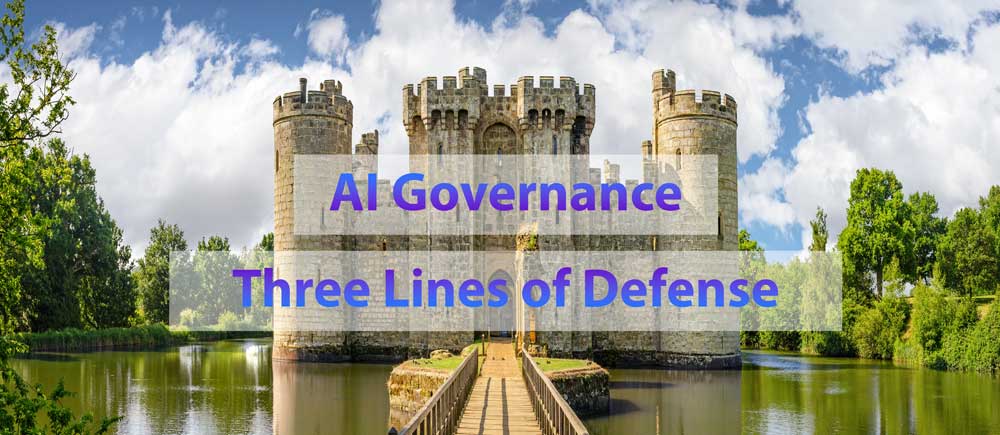
The Three “Lines of Defense” Every ML Program Needs: AI Governance
As Artificial Intelligence and Machine Learning creep further and further into our businesses and everyday lives, it’s important that we take stock on thoughtful, ethical, and responsible execution and strategy of AI/ML.
On the business side, more and more businesses are launching “Digital Transformation” and “Innovation” initiatives, groups, and executive roles to level-up these nascent AI/ML programs on the shoulders of scaleable AI platforms. On the public side, the impacts are quite different and can be somewhat eye-opening.
Recently, Twitter admitted to having bias in their algorithm towards right-wing news. Even Apple experienced gender bias in their credit card, giving males a higher credit limit than females with the same attributes in the same household. With the great promise of AI, comes great responsibility, and it’s very important to have multiple lines of defense to position against blemishes such as these. These lines of defense in machine learning are called AI Governance. Even in sports like football, there are three lines of defense against long-term and permanent injury. For example, there are rules and the officials that enforce them, the safety equipment (pads) that the athletes wear, and lastly, there are medical professionals on the sideline to tend to any injuries. All of these in concert contribute to not just a safe game, but a game that is more enjoyable by fans and hence profitable for the business of football.
So, What Are the Three “Lines of Defense” in ML?
1st Line of Defense – Data scientists (DS) build models in the lab using training data and will use different tools and techniques to create a first line of defense to ensure they can detect issues such as accuracy, precision, bias, drift. This allows the data scientists the best chance to ensure their models do not create issues before these models leave the lab environment. Once these models leave the lab, data scientists generally don’t have a purview into the performance of models in production when real-world data is used as the input and customers encounter these outputs.
2nd Line of Defense – Once models are completed by the data scientist, they are handed over to the IT side of the house where a DevOps engineer or ML engineer deploys the model into “production.” Only in production does the model have the opportunity to deliver true value to the business, and 90% of ML models never make it into production. Even though data scientists have done a good job in the 1st line of defense to ensure models are void of issues, data encountered in production will very often be different from what was done in the lab. This is why the second line of defense is so important. The second line of defense sits upon the DevOps engineer or ML engineer to employ a mechanism to cross-check what the data scientists have done in a production environment; re-confirming what the data scientist did in the lab does not deviate in production, and if they deviate there is a feedback loop to collaborate and correct. Additionally, this includes monitoring the performance of the model to ensure it is functioning properly from an operational perspective.
3rd Line of Defense – Companies that are just starting out on AI projects may not factor in Risk and Compliance as an element, because they neither have the resources nor are they considered critical to the business. However, as their AI projects get traction, these teams become instrumental to guard against unnecessary exposure to risk and cavalier decision-making. As AI/ML programs are still in their relative infancy, there are few guard rails in place and government regulation is still being formulated, hence this responsibility is left upon the practitioner, business, and in worst cases, the press or government. Wanting to avoid PR issues and government intervention and fines, businesses develop Risk and Compliance teams to self-regulate. The third line of defense for AI/ML programs entails Risk & Compliance teams auditing potentially thousands of models and understanding how the models are performing in a business context (bias, drift, alerts, model changes “audit log,” custom metrics, explainability). Regardless of whether or not you have a Risk and Compliance requirement, AI/ML business unit executives need to be able to explain exactly what their models are doing in production and ensure safeguards are in place through proper reporting. It is the BU or LOB leader who is the last line of defense and is responsible for the behavior and performance of the AI/ML program. They cannot solely rely on the data scientists or DevOps/ML engineers as a safety layer between the organization and the real world.
Conclusion: AI Governance is Mandatory for AI Programs of All Sizes
To conclude, as practitioners in the AI/ML space, it is all of our responsibility to shepherd this technology into existence reliably, ethically, and responsibly, employing an AI Governance framework. Being an early or small AI/ML program is no excuse for not employing all three lines of defense. In the end, all three practitioners (data scientists, IT, and executives) are responsible for the overall health, performance, and safety of the AI/ML program. All practitioners should seek out solutions that build an impenetrable fortress to protect their program, the business, and the promise of AI for humanity.
About Datatron – The AI Governance & MLOps Platform
Datatron is a leader in helping enterprises build a robust AI Governance solution with multiple Fortune 1000 clients successfully running models in production. With Datatron, data scientists can now monitor their models in production in real-time for bias, drift, and performance anomalies. DevOps engineers and ML engineers can receive alerts when issues arise before they become problems. And business leaders and Risk and Compliance teams can receive audit reports that explain exactly what models are doing in production. Datatron is a shield for your AI/ML program with features for all three lines of defense. Book a Demo to learn governance best practices.