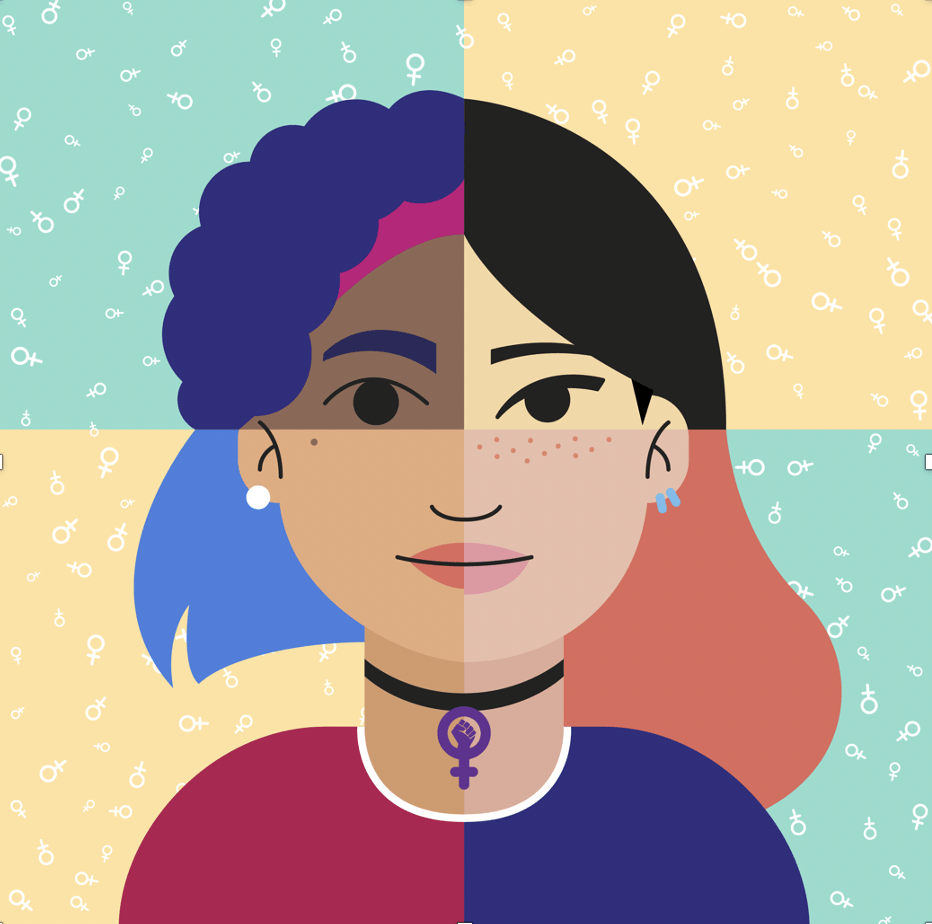
Real-life Examples of Discriminating Artificial Intelligence
Artificial Intelligence.
Some say that it’s a buzzword that doesn’t really mean much. Others say that it’s the cause of the end of humanity.
The truth is that artificial intelligence (AI) is starting a technological revolution, and while AI has yet to take over the world, there’s a more pressing concern that we’ve already encountered: AI bias.
What is AI bias?
AI bias is the underlying prejudice in data that’s used to create AI algorithms, which can ultimately result in discrimination and other social consequences.
Let me give a simple example to clarify the definition: Imagine that I wanted to create an algorithm that decides whether an applicant gets accepted into a university or not and one of my inputs was geographic location. Hypothetically speaking, if the location of an individual was highly correlated with ethnicity, then my algorithm would indirectly favor certain ethnicities over others. This is an example of bias in AI.
This is dangerous. Discrimination undermines equal opportunity and amplifies oppression. I can say this for certain because there have already been several instances where AI bias has done exactly that.
In this article, I’m going to share three real-life examples of when AI algorithms have demonstrated prejudice and discrimination towards others.
Three Real-Life Examples of AI Bias
1. Racism embedded in US healthcare
In October 2019, researchers found that an algorithm used on more than 200 million people in US hospitals to predict which patients would likely need extra medical care heavily favored white patients over black patients. While race itself wasn’t a variable used in this algorithm, another variable highly correlated to race was, which was healthcare cost history. The rationale was that cost summarizes how many healthcare needs a particular person has. For various reasons, black patients incurred lower healthcare costs than white patients with the same conditions on average.
Thankfully, researchers worked with Optum to reduce the level of bias by 80%. But had they not been interrogated in the first place, AI bias would have continued to discriminate severely.
2. COMPAS
Arguably the most notable example of AI bias is the COMPAS (Correctional Offender Management Profiling for Alternative Sanctions) algorithm used in US court systems to predict the likelihood that a defendant would become a recidivist.
Due to the data that was used, the model that was chosen, and the process of creating the algorithm overall, the model predicted twice as many false positives for recidivism for black offenders (45%) than white offenders (23%).
3. Amazon’s hiring algorithm
Amazon’s one of the largest tech giants in the world. And so, it’s no surprise that they’re heavy users of machine learning and artificial intelligence. In 2015, Amazon realized that their algorithm used for hiring employees was found to be biased against women. The reason for that was because the algorithm was based on the number of resumes submitted over the past ten years, and since most of the applicants were men, it was trained to favor men over women.
What can we learn from all of this?
It’s clear that making non-biased algorithms are hard. In order to create non-biased algorithms, the data that’s used has to be bias-free and the engineers that are creating these algorithms need to make sure they’re not leaking any of their own biases. With that said, here are a few tips to minimize bias:
1.The data that one uses needs to represent “what should be” and not “what is”
. What I mean by this is that it’s natural that randomly sampled data will have biases because we lived in a biased world where equal opportunity is still a fantasy. However, we have to proactively ensure that the data we use represents everyone equally and in a way that does not cause discrimination against a particular group of people. For example, with Amazon’s hiring algorithm, had there been an equal amount of data for men and women, the algorithm may not have discriminated as much.
2.Some sort of data governance should be mandated and enforced
. As both individuals and companies have some sort of social responsibility, we have an obligation to regulate our modeling processes to ensure that we are ethical in our practices. This can mean several things, like hiring an internal compliance team to mandate some sort of audit for every algorithm created, the same way Obermeyer’s group did.
3.Model evaluation should include evaluation by social groups
. Learning from the instances above, we should strive to ensure that metrics like the true accuracy and false positive rate are consistent when comparing different social groups, whether that be gender, ethnicity, or age.
What else do you guys think? What are some best practices that everyone should conduct to minimize AI bias! Leave a comment and let’s discuss!
Here at Datatron, we offer a platform to govern and manage all of your Machine Learning, Artificial Intelligence, and Data Science Models in Production. Additionally, we help you automate, optimize, and accelerate your ML models to ensure they are running smoothly and efficiently in production — To learn more about our services be sure to Book a Demo.